Google announces 5 safety rules for its artificial intelligence development
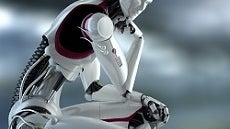
Every year without fail, Google promotes the depth and breadth of its “machine learning” prowess at its various developer gatherings, most notably, Google I/O.
Progress in this area of is proceeding in directions that are still being discovered. When you couple that reality with Hollywood portrayals from the benevolent, like Star Trek’s “computer,” to the mission-conflicted, like 2001: A Space Odyssey’s HAL9000, to the self-aware, humanity-destroying Skynet from Terminator, it raises genuine questions about how to address practical problems, and prevent accidents in the real-world AI systems.
Chris Olah at Google Research contributed to a technical paper, Concrete Problems in AI Safety, in collaboration with Google, OpenAI, Stanford University, and University of California, Berkeley, to define how to approach long-term research questions:
These questions, not unlike The Three Laws of Robotics, should provide some meaningful guidance as we see the products of AI and machine learning find their way ever-more-present in consumer applications. Google Home, Amazon Echo, Apple’s HomeKit, those platforms will be the most immediately visible platforms to the consumer, supplemented by smarter vehicles, managed by our smartphones, and many other things we have still yet to think of.
What do you think of these researchers’ visions for AI development?
sources: Google Research via CNET
Progress in this area of is proceeding in directions that are still being discovered. When you couple that reality with Hollywood portrayals from the benevolent, like Star Trek’s “computer,” to the mission-conflicted, like 2001: A Space Odyssey’s HAL9000, to the self-aware, humanity-destroying Skynet from Terminator, it raises genuine questions about how to address practical problems, and prevent accidents in the real-world AI systems.
Avoiding Negative Side Effects: How can we ensure that an AI system will not disturb its environment in negative ways while pursuing its goals, e.g. a cleaning robot knocking over a vase because it can clean faster by doing so?
Avoiding Reward Thinking: How can we avoid gaming of the reward function? For example, we don’t want this cleaning robot simply covering over messes with materials it can’t see through.
Safe Exploration: How do we ensure that an AI system doesn’t make exploratory moves with very negative repercussions? For example, maybe a cleaning robot should experiment with mopping strategies, but clearly it shouldn’t try putting a wet mop in an electrical outlet.
Robustness to Distributional Shift: How do we ensure that an AI system recognizes, and behaves robustly, when it’s in an environment very different from its training environment? For example, heuristics learned for a factory workfloor may not be safe enough for an office.
Scalable Oversight: How can we efficiently ensure that a given AI system respects aspects of the objective that are too expensive to be frequently evaluated during training? For example, if an AI system gets human feedback as it performs a task, it needs to use that feedback efficiently because asking too often would be annoying.
Safe Exploration: How do we ensure that an AI system doesn’t make exploratory moves with very negative repercussions? For example, maybe a cleaning robot should experiment with mopping strategies, but clearly it shouldn’t try putting a wet mop in an electrical outlet.
Robustness to Distributional Shift: How do we ensure that an AI system recognizes, and behaves robustly, when it’s in an environment very different from its training environment? For example, heuristics learned for a factory workfloor may not be safe enough for an office.
These questions, not unlike The Three Laws of Robotics, should provide some meaningful guidance as we see the products of AI and machine learning find their way ever-more-present in consumer applications. Google Home, Amazon Echo, Apple’s HomeKit, those platforms will be the most immediately visible platforms to the consumer, supplemented by smarter vehicles, managed by our smartphones, and many other things we have still yet to think of.
What do you think of these researchers’ visions for AI development?
sources: Google Research via CNET
Things that are NOT allowed:
To help keep our community safe and free from spam, we apply temporary limits to newly created accounts: